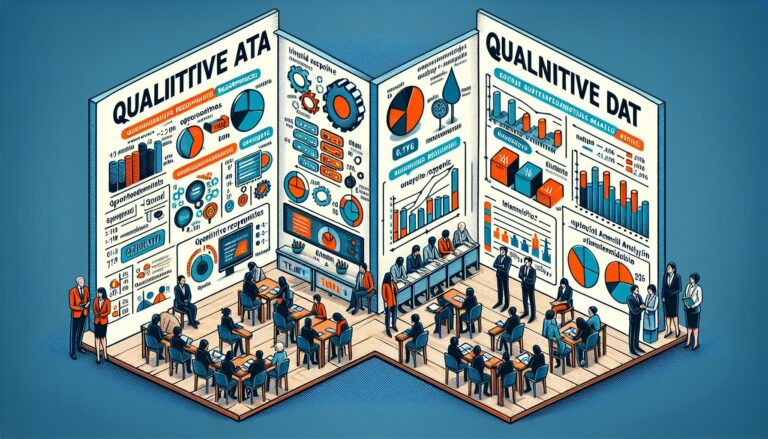
Difference Between Qualitative Data and Quantitative lies in their nature and application. Qualitative data is descriptive and subjective, focusing on themes and insights, while quantitative data is numerical and objective, emphasizing measurement and statistical analysis for validation.
Understanding the Basics of Qualitative and Quantitative Data
When delving into research methodology, it’s crucial to grasp the difference between qualitative data and quantitative data. Qualitative data focuses on the nuances, capturing the essence of experiences, opinions, and motivations. This type of data is often descriptive, with insights derived from interviews, open-ended surveys, and observations. It helps convey the complexity of human behavior and social phenomena, allowing researchers to form deeper connections with their subjects. Common characteristics of qualitative data include:
- Subjectivity: Driven by personal perspectives and context.
- Richness: Offers detailed insights that simple numbers can’t express.
- Contextual Understanding: Captures the environment and circumstances surrounding data points.
In contrast, quantitative data is numeric and can be statistically analyzed. It provides measurable and comparable results, commonly obtained through structured surveys, experiments, and existing datasets. This form of data is invaluable for establishing generalizable patterns and testing hypotheses. Typically, quantitative data is characterized by:
- Objectivity: Minimizes personal biases and focuses on measurable outcomes.
- Statistical Analysis: Enables researchers to apply various tests to validate findings.
- Generalizability: Allows for broader conclusions across larger populations.
In examining the difference between qualitative data and quantitative, one can better determine the appropriate approach for a given research question, balancing rich, narrative insights with robust, statistical findings.
Aspect | Qualitative Data | Quantitative Data |
---|---|---|
Nature | Descriptive | Numeric |
Analysis | Thematic | Statistical |
Outcome | Insights | Patterns |
Understanding the difference between qualitative data and quantitative data allows researchers to deploy the right methods and ultimately achieve their research goals more effectively.
Key Characteristics of Qualitative Data
Qualitative data is distinguished by its rich, narrative form, providing insights into human behavior, attitudes, and experiences. Unlike quantitative data, which presents information in numerical values, qualitative data captures the essence of subjective experiences through methods such as interviews, focus groups, or open-ended surveys. This intrinsic nature enables researchers and analysts to explore complex phenomena, revealing patterns and themes that may not be apparent in a purely numerical analysis. include:
- Descriptive: It encompasses words, images, or narratives, allowing for a deeper understanding of context.
- Contextual: It emphasizes the social and cultural contexts of the subjects being studied.
- Subjective: It relies on individual perceptions and interpretations, making the findings more personal and less standardized.
- Exploratory: It is often used in the initial stages of research to identify patterns or generate hypotheses.
The difference between qualitative data and quantitative data can be visually summarized through the following table, illustrating their contrasting features:
Qualitative Data | Quantitative Data |
---|---|
Descriptive analysis | Statistical analysis |
Subjective interpretations | Objective measurements |
Open-ended responses | Closed-ended responses |
By understanding these key characteristics, we begin to see the difference between qualitative data and quantitative data in practice, particularly in how they inform research outcomes and influence decision-making processes. Qualitative data is invaluable for its ability to delve into the feelings, motivations, and social contexts of individuals, whereas quantitative data serves to quantify trends, providing a broader but potentially less nuanced perspective. Recognizing these distinctions enhances the ability to choose the appropriate methodology based on the research goals, emphasizing the significance of the difference between qualitative data and quantitative data in generating comprehensive insights.
Key Characteristics of Quantitative Data
Quantitative data is characterized by its reliance on numerical values and statistical analysis, allowing for measurable and objective conclusions. This type of data is ideal for identifying patterns, making predictions, and testing hypotheses due to its ability to quantify phenomena. Some of the include:
- Measurable Values: Quantitative data can be counted or measured, providing tangible metrics for analysis.
- Statistical Analysis: This data type enables the use of various statistical methods, making it essential for rigorous research.
- Consistency: Quantitative data is often collected in a standardized manner, ensuring reliability and validity across different studies.
To further illustrate the significance of quantitative data in understanding the difference between qualitative data and quantitative, consider its application in surveys or experiments. For example, the following table compares numerical responses versus open-ended responses gathered in a research study:
Data Type | Example of Data Collected | Purpose |
---|---|---|
Quantitative | Rating from 1 to 10 | Measure satisfaction levels |
Qualitative | Open-ended text response | Gather detailed opinions |
By comparing these two categories, one can clearly see the difference between qualitative data and quantitative. Quantitative data provides the hard evidence necessary for empirical analysis, making it a cornerstone of scientific research and data-driven decision-making. In contrast, qualitative data offers rich insights that are crucial for context and understanding, but it lacks the quantifiable nature found in quantitative analysis.
Comparative Analysis of Data Collection Methods
When exploring the difference between qualitative data and quantitative, it is essential to recognize the various data collection methods employed in each approach. Qualitative data collection typically involves methods that provide rich, in-depth insights and understanding of human behavior and experiences. Common techniques include:
- Interviews: Engaging with participants to extract personal narratives and perceptions.
- Focus Groups: Facilitating discussions among diverse participants to generate collective insights.
- Observations: Noting behaviors and interactions in natural settings to gather contextual information.
Conversely, quantitative data emphasizes measurement and numerical analysis, prioritizing statistical conclusions over subjective interpretations. The methods for collecting quantitative data are designed to yield measurable and comparable results. These techniques include:
- Surveys: Utilizing structured questionnaires with fixed responses to gather data from a larger audience.
- Experiments: Conducting controlled studies to manipulate variables and observe outcomes.
- Secondary Data Analysis: Analyzing existing datasets to find patterns and trends.
Recognizing the difference between qualitative data and quantitative not only enhances data collection strategies but also informs the selection of the appropriate methodology based on research objectives. Both methods provide unique insights, and understanding their nuances allows researchers to choose the best approach for their specific inquiries.
The Role of Subjectivity in Qualitative Research
In qualitative research, subjectivity plays a pivotal role by allowing researchers to delve deep into the human experience, capturing feelings, thoughts, and interpretations that quantitative methods might overlook. This approach often involves the researcher’s own perspectives and biases, which can enrich the data collected but also necessitate a careful consideration of how these subjective viewpoints influence the findings. By embracing this subjectivity, qualitative research offers insights that are nuanced and contextually relevant, providing a contrast to the more detached nature of quantitative data collection. The richness of qualitative data stems from its ability to communicate complex human emotions and social contexts, showcasing a difference between qualitative data and quantitative that is stark and significant.
This interaction between researcher and participant can be seen in various qualitative methodologies, such as interviews, focus groups, and ethnography. Here, the deep engagement often reveals unexpected themes and patterns, showcasing how subjective experiences can lead to a deeper understanding of a phenomenon. In stark opposition, quantitative research generally relies on numerical data and statistical analysis, minimizing the researcher’s influence and emphasizing objectivity. This fundamental difference between qualitative data and quantitative approaches highlights how qualitative research can uncover the layered intricacies of human life, allowing for a more comprehensive exploration of social phenomena that pure numbers often fail to convey.
The Role of Objectivity in Quantitative Research
In the realm of quantitative research, objectivity is the cornerstone that separates rigorous scientific inquiry from subjective interpretation. It ensures that the data collected reflects the true reality being studied rather than the biases of the researcher. The emphasis on objectivity mandates the use of standardized tools and methods for data collection and analysis, minimizing the potential for personal bias to skew results. This is crucial in establishing the difference between qualitative data and quantitative approaches, as quantitative methods focus on measurable variables, leaving little room for personal interpretation. By adhering to strict protocols, researchers can achieve greater reliability and validity in their findings.
Furthermore, the objectivity inherent in quantitative research allows for broader generalizations from a sample to a larger population. It enables researchers to apply statistical techniques, yielding numerical data that can be manipulated for insightful analysis. When exploring the difference between qualitative data and quantitative, it’s evident that while qualitative data delves into the “why” and “how” behind behaviors, quantitative data quantifies these insights for a clearer picture of the trends. The use of tools such as surveys or experiments reinforces the empirical nature of quantitative research, making objectivity not just a guideline, but a fundamental principle that enhances the credibility of the study.
Choosing the Right Data Type for Your Research Goals
When undertaking research, understanding the difference between qualitative data and quantitative data is crucial for aligning your methodologies with your research objectives. Qualitative data focuses on the depth of understanding and explores the nuances within human experience, often employing interviews, focus groups, and open-ended surveys to gather rich narratives. This data type excels in capturing complexity, providing insights into behaviors, motivations, and emotions, which can lead to the development of theories and concepts. In contrast, quantitative data emphasizes numerical measurement and statistical analysis, making use of structured surveys and experiments to ensure that results can be generalized across broader populations.
Ultimately, the choice between these data types hinges on what you aim to achieve. If your goal is to explore intricate social phenomena, understanding context, and capturing subjective experiences, qualitative methods may serve you best. However, if you are interested in testing a hypothesis, measuring variables, or establishing patterns, a quantitative approach will provide the precision and reliability you need. By clarifying your research goals, you can make an informed decision regarding which data type to employ, keeping in mind the difference between qualitative data and quantitative approaches and their respective strengths.
Combining Qualitative and Quantitative Data: A Mixed Methods Approach
Combining qualitative and quantitative data enables researchers to leverage the strengths of both methodologies, leading to a more comprehensive understanding of complex phenomena. The difference between qualitative data and quantitative data lies primarily in their nature. Qualitative data is descriptive and often collected through interviews, observations, or open-ended surveys, focusing on the depth and context of human behavior. In contrast, quantitative data is numerical and statistical, derived from tools such as structured surveys and experiments that allow for measurable results. By blending these two forms of data, researchers can enrich their findings to not only identify patterns but also understand the nuances behind them.
In practice, a mixed methods approach can be illustrated through a simple research study on consumer behavior. For instance, researchers might first gather qualitative insights through focus groups to explore why customers prefer specific brands. They could then follow this with a quantitative survey to measure how many customers share these preferences and the statistical significance of the findings. The difference between qualitative data and quantitative data is underscored as the qualitative data provides context for the numbers that follow, allowing for a narrative that explains the quantitative results. employing both data types in tandem results in richer, more actionable insights that a single method alone could never yield.
Aspect | Qualitative Data | Quantitative Data |
---|---|---|
Nature | Descriptive | Numerical |
Collection Methods | Interviews, Observations | Surveys, Experiments |
Focus | Depth | Statistics |
Objective | Understanding Context | Measuring Impact |
Interpreting Findings: Insights from Qualitative vs. Quantitative Data
When delving into the Difference Between Qualitative Data and Quantitative data, it’s essential to recognize how these contrasting approaches yield unique insights. Qualitative data offers a rich, nuanced understanding of human behaviors and emotions, often captured through interviews, focus groups, and open-ended surveys. This form of data reveals the underlying motivations and feelings that drive people’s actions, providing context that numbers alone cannot convey. For example, themes derived from qualitative analyses can highlight common challenges faced by individuals, offering a deeper look into the societal factors at play.
In contrast, quantitative data thrives on measurable statistics and numerical assessments, allowing researchers to identify broader patterns across large populations. This form of data can be gathered through structured surveys, experiments, and observations that result in numerical outputs, which can then be analyzed using various statistical methods. The Difference Between Qualitative Data and Quantitative lies in their methods—while qualitative focuses on ‘why’ and ‘how’, quantitative emphasizes ‘what’ and ‘how many’. To illustrate these differences, the following table summarizes key characteristics:
Aspect | Qualitative Data | Quantitative Data |
---|---|---|
Data Type | Descriptive | Numerical |
Collection Methods | Interviews, Focus Groups | Surveys, Experiments |
Analysis | Thematic Analysis | Statistical Analysis |
Outcome | Insights & Emotions | Trends & Patterns |
This dichotomy emphasizes that understanding the Difference Between Qualitative Data and Quantitative data is crucial for researchers aiming to craft a comprehensive view of their subject matter. Both approaches are invaluable, and when utilized together, they can enrich the analysis, providing a holistic view that can address both statistical trends and personal narratives. By leveraging the strengths of each type of data, one can generate insights that are both grounded in evidence and attuned to the human experience.
Common Mistakes in Data Analysis and How to Avoid Them
When analyzing data, one common mistake is blending qualitative and quantitative data without a clear understanding of its implications. The difference between qualitative data and quantitative information lies in their nature; qualitative data is descriptive and subjective, while quantitative data is numerical and objective. By failing to recognize these differences, analysts may misinterpret findings, leading to erroneous conclusions. To avoid this, it is crucial to establish a framework that clearly defines the types of data being manipulated and the appropriate methods of analysis for each. Utilize checks like data type validation and maintain clear documentation on the expected outcomes from both qualitative and quantitative analyses.
Another common pitfall is assuming that all data can be analyzed using the same statistical techniques. The difference between qualitative data and quantitative formats necessitates different analytical approaches. For instance, attempting to perform regression analysis on qualitative data, which often involves categorical variables, can skew results and misguide decision-making. To mitigate this, analysts should adopt appropriate research methodologies tailored to the data type. Consider using methods like thematic analysis for qualitative data and descriptive statistics for quantitative data. This approach helps maintain clarity and rigor in the analytic process and prevents misinterpretations that could arise from conflating the two data types.
Best Practices for Collecting Qualitative and Quantitative Data
Collecting qualitative and quantitative data effectively requires a careful blend of strategy, methodology, and adaptability. For qualitative data, some best practices include conducting in-depth interviews, utilizing focus groups, and employing open-ended surveys. These methods allow for rich, detailed insights that capture the nuances of human experience and opinion, which is essential for understanding the difference between qualitative data and quantitative. Ensuring that interviewers are trained to ask probing questions and encourage participants to share their thoughts freely can yield more informative responses. Additionally, using transcription software can help organize these insights for further analysis.
When it comes to quantitative data collection, employing structured surveys with closed-ended questions is imperative. Online tools like Google Forms or SurveyMonkey can enhance accessibility and convenience for respondents. It’s crucial to define your metrics clearly, as this will make distinguishing the difference between qualitative data and quantitative more straightforward. To visualize the results, consider using data visualization tools that offer charts or graphs. Below is a simple table showcasing different methods of data collection and their respective strengths:
Data Collection Method | Type of Data | Strengths |
---|---|---|
Interviews | Qualitative | In-depth insights, personal stories |
Surveys | Quantitative | Large sample size, easy analysis |
Focus Groups | Qualitative | Diverse perspectives, live feedback |
Statistical Analysis | Quantitative | Data-driven conclusions, trends |
Future Trends in Qualitative and Quantitative Research Techniques
As we look towards the future, the landscape of qualitative and quantitative research is poised for significant evolution driven by technological advancements and changing research needs. The difference between qualitative data and quantitative techniques is becoming increasingly blurred as researchers seek innovative ways to merge both paradigms. For instance, the integration of artificial intelligence and machine learning not only enhances data analysis but also allows researchers to gather and interpret qualitative insights at an unprecedented scale. Tools like sentiment analysis and automated coding software are paving the way for richer qualitative datasets, while maintaining the rigor traditionally associated with quantitative measures.
Furthermore, the rise of big data is transforming how both qualitative and quantitative research is conducted. Researchers now have access to vast amounts of unstructured data from social media, online surveys, and other digital platforms, which invites new methodologies that leverage both qualitative insights and quantitative analytics. The difference between qualitative data and quantitative methods is starting to dissolve, giving birth to hybrid approaches that yield comprehensive insights. This shift not only enhances the effectiveness of research findings but also encourages a collaborative environment where mixed-method research thrives, ultimately enriching our understanding of complex social phenomena.
Frequently Asked Questions
Q&A: Unraveling the Differences Between Qualitative and Quantitative Data
Q1: What is the primary distinction between qualitative and quantitative data?
A1: At its core, qualitative data is descriptive and conceptual, focusing on understanding the qualities or characteristics that shape human experiences and perspectives. It encompasses non-numeric information, such as interviews, open-ended responses, and observations. In contrast, quantitative data is all about measurement and numbers, offering a way to quantify characteristics, behaviors, or variables. This type of data is often gathered through structured methods like surveys with fixed responses or experiments that produce measurable outcomes.
Q2: Can you provide examples of qualitative data?
A2: Certainly! Examples of qualitative data include descriptive accounts from focus groups discussing a new product, narrative responses in interviews that explore customer satisfaction, or observational notes detailing interactions in a natural setting. Think of it as exploring the “why” and ”how” behind human feelings, motivations, and behaviors.
Q3: And what about quantitative data? What does that look like?
A3: Quantitative data is characterized by its numerical nature. Examples include statistical information like the number of customers who purchased a product, survey results with rating scales, or measurements of performance metrics. It answers questions like ”how much,” “how often,” or “to what extent” with clear, measurable values. This type of data is typically analyzed using statistical methods to identify patterns or correlations.
Q4: When should researchers use qualitative data over quantitative data, and vice versa?
A4: The choice between qualitative and quantitative data largely depends on the research objectives. If the goal is to gain deep insights into complex behaviors, perceptions, or emotions, qualitative data is invaluable. Researching phenomena like customer satisfaction or user experiences often relies on qualitative approaches. On the other hand, if the objective is to test a hypothesis, measure variables, or generalize findings across a larger population, quantitative data is the preferred choice. It enables researchers to analyze trends and make predictions based on numerical trends.
Q5: Is it possible to combine qualitative and quantitative data in research?
A5: Absolutely! This approach, known as mixed-methods research, allows researchers to capitalize on the strengths of both types of data. For example, one might conduct a quantitative survey to identify general trends in customer behavior and follow up with qualitative interviews to delve deeper into those trends and understand the underlying motivations. Combining both methods provides a more comprehensive understanding of research questions and adds robustness to the findings.
Q6: What are some common misconceptions about qualitative and quantitative data?
A6: One common misconception is that qualitative data is inherently less rigorous than quantitative data. In reality, both types of data have their own strengths and challenges, and rigor can be found in both approaches. Another misconception is that qualitative data cannot be analyzed statistically; while it is true that the analysis methods differ, qualitative data can still be coded and quantified to some extent, allowing for structured analysis.
Q7: How can someone new to research begin to navigate the world of qualitative and quantitative data?
A7: A good starting point is to familiarize oneself with basic research concepts and methodology. Online courses or workshops can provide valuable insight into data collection techniques, analysis methods, and interpretation of results. Additionally, reading case studies that highlight the use of both types of data in real-world research can help illuminate their practical applications. Most importantly, remain curious and open to exploring both worlds, as each offers unique perspectives that can enhance our understanding of complex issues.
By fostering a deeper understanding of both qualitative and quantitative data, researchers can enhance their approach and ultimately make more informed decisions in their studies. Each type of data plays its own vital role in the expansive landscape of research.
Insights and Conclusions
understanding the Difference Between Qualitative Data and Quantitative is essential for researchers and analysts alike. While qualitative data provides depth and context, quantitative data offers measurable insights. Recognizing the Difference Between Qualitative Data and Quantitative helps in choosing the right approach for effective data analysis and interpretation.